The big risk of big data
One of the benefits of the 21st century is the availability of large amounts of data that can be analysed quickly. Big data, in combination with AI, has the potential to revolutionise the investment industry – as well as ruin it. After all, the financial industry is famous for reinventing the wheel in ever more complex and complicated versions – just to see this new wheel break down when it hits the first pothole. In my experience, big data and AI have so far not produced any meaningful progress in investment processes and the returns generated by new algorithms that rely on big data have been virtually indistinguishable from returns generated by more traditional investment approaches. Now that may change in the future, but it won’t be easy.
One of the challenges for big data applications is that with more data it is getting easier and easier to overfit a model. And this leads to trading or investment strategies that look great in backtests but perform poorly out of sample. David Bailey and Marcos Lopez de Prado have shown in an article for the Journal of Portfolio Management how overfitting can create false positives in large data sets and how the Sharpe ratio of a model backtest needs to be deflated in order to assess the true potential of a strategy.
One example, where the effects of overfitting have been made amply clear is in the research of Campbell Harvey and his colleagues on factors that explain the cross-section of equity returns. Smart beta and factor investing have become the main trend for institutional investors in recent years, though the results are sometimes not what investors expected. In his research, Campbell Harvey showed that many factors that were identified as statistically significant in the literature were likely the result of data mining and data snooping. As is so often the case, it seems that some factors may have been yet another wheel that broke down at the first pothole. However, there are some factors like the momentum and the value factor that seem to be true factors and above suspicion of data mining.
But how good does an investment strategy have to be to be above suspicion? David Bailey provided the chart below that gives some guidance. On the horizontal axis, the chart shows, how often a strategy has been tested. This can be if you test a strategy with different parameters (e.g. the length of a moving average) on the same historical data, or you use different backtesting periods or data sets for the same strategy (e.g. testing a strategy for all the individual stocks in the S&P 500). Bailey’s chart shows that even if the strategy you are testing is useless and has a true Sharpe ratio of 0, the likelihood of getting a Sharpe ratio of 3 or more is pretty high. In Bailey’s chart, the horizontal axis plots the number of backtests, while the vertical axis plots the distribution of Sharpe ratios of the different backtests. The colour in the chart shows how likely it is to get that result with brighter colours indicating a higher likelihood and the dashed line shows the expected maximum Sharpe ratio you should see in your many trials. If one runs 1,000 backtests, one would expect to see at least one strategy that creates a Sharpe ratio of 3.26.
Now imagine the fantastic results one can get by exploring big data and running millions of tests for investment strategies. One is almost bound to find strategies with a Sharpe ratio of 5! And such a strategy would almost inevitably be promoted as the next big thing, attracting a large amount of investments – and then failing to deliver on its promises. It seems to me that very few institutions that investigate the possibilities of big data and AI in finance are aware of the size of these false signals. And I am pretty sure most investors aren’t aware of it either, making them easy prey for yet another disastrous investment product in the future.
The maximum Sharpe ratio expected from a random strategy
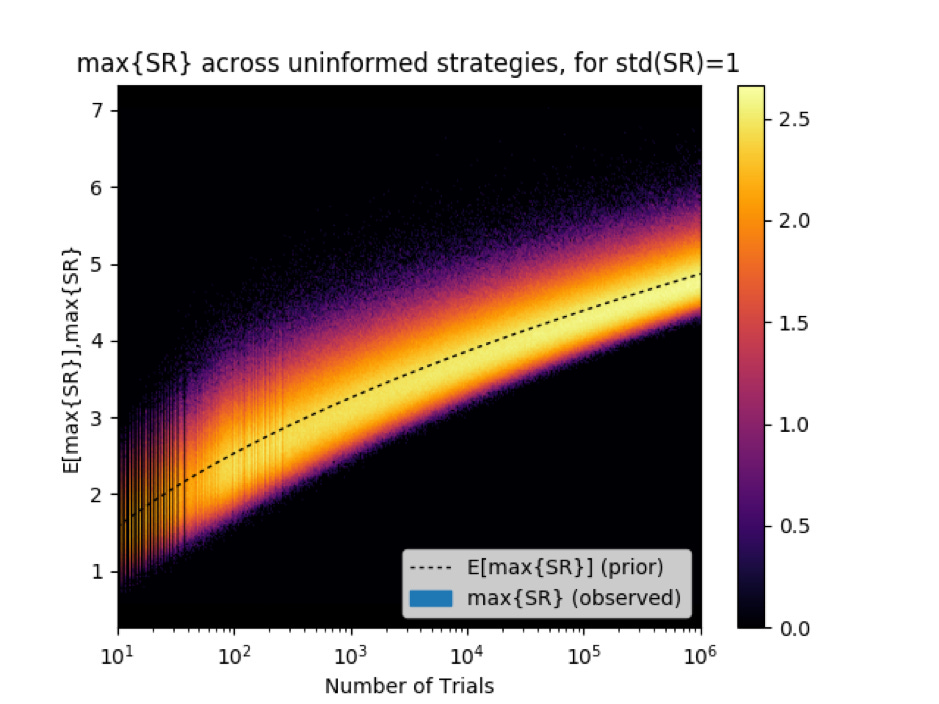
Source: Mathematical Investor.